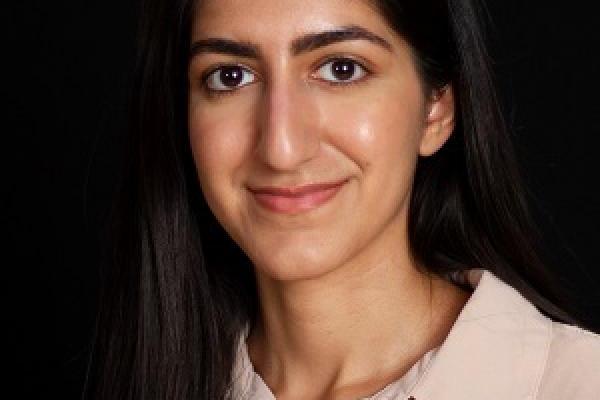
Dissertation Defense: Pashmeen Kaur, Statistics Ph.D. Candidate
Title: Statistical Methods for Generalized Integer Autoregressive Processes
Abstract: A popular and flexible time series model for counts is the generalized integer autoregressive process of order p, GINAR(p). These Markov processes are defined using thinning operators evaluated on past values of the process along with a discretely-valued innovation process. This class includes the commonly used INAR(p) process, defined with binomial thinning and Poisson innovations. GINAR processes can be used in a variety of settings, including modeling time series with low counts, and allow for more general mean-variance relationships, capturing both over- or under-dispersion.
While there are many thinning operators and innovation processes given in the literature, less focus has been spent on comparing statistical inference and forecasting procedures over different choices of GINAR process. We provide an extensive study of exact and approximate inference and forecasting methods that can be applied to a wide class of GINAR(p) processes with general thinning and innovation parameters. We discuss the challenges of exact estimation when p is larger. We summarize and extend asymptotic results for estimators of process parameters, and present simulations to compare small sample performance, highlighting how different methods compare.
GINAR processes assume stationarity of the process, which may not be an appropriate assumption for many real-world applications. Hence, we introduce a process for non-stationary count time series called the time-varying generalized integer autoregressive process (TV-GINAR(p)), which allows for time-varying parameters modeled via basis functions. We introduce statistical properties, discuss estimation strategies, and statistical inference for this class of processes. We present simulation studies for the TV-GINAR(p) process and illustrate this methodology by fitting GINAR and TV-GINAR processes to a disease surveillance series and patient scores dataset.
Advisor: Peter Craigmile
Zoom Link: https://osu.zoom.us/j/98197337721?pwd=6FbwyW6bHeFlo88Of0K38e74yXuqWA.1