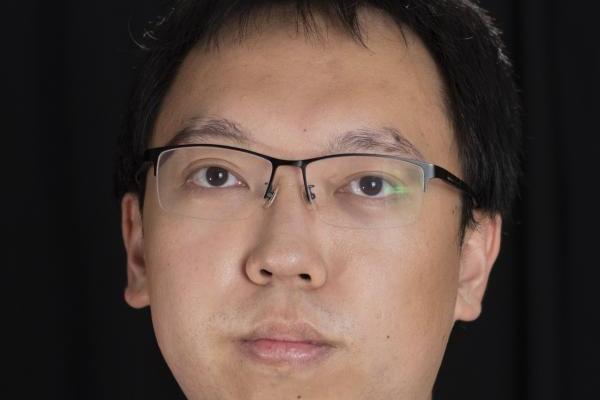
Dissertation Defense: Rui Qiang, Statistics Ph.D. Candidate
Title: Accounting for Preferential Sampling in Geostatistical Inference
Abstract: In geostatistical inference, preferential sampling takes place when the locations of point-referenced data are related to the latent spatial process of interest. Traditional geostatistical models can lead to biased inferences and predictions under preferential sampling. We introduce an extended Bayesian hierarchical framework that models both the observation locations and the observed data jointly, using a spatial point process for the locations and a geostatistical process for the observations. We first develop a hierarchical model using a log-Gaussian Cox process (LGCP) for the sampling locations, combined with a Gaussian process for the observations. This model is extended to incorporate non-Gaussian observations through spatial generalized linear models (GLMs), adding flexibility to this preferential sampling framework. To reduce computational complexity, we adopt techniques like nearest neighbor approximation. We also introduce simpler methods for accounting for preferential sampling that are less computationally demanding at the expense of prediction accuracy. We validate our models through extensive simulations, demonstrating their effectiveness in correcting biases and improving prediction accuracy. We apply our models to decadal average temperature data from the Global Historical Climate Network in Southwestern United States and show that preferential sampling could be present in California.
Advisor: Peter Craigmile
Zoom Link: https://osu.zoom.us/j/96876737733?pwd=deU0xXseFKbaDDlzYtMpTJ9dZITrVJ.1