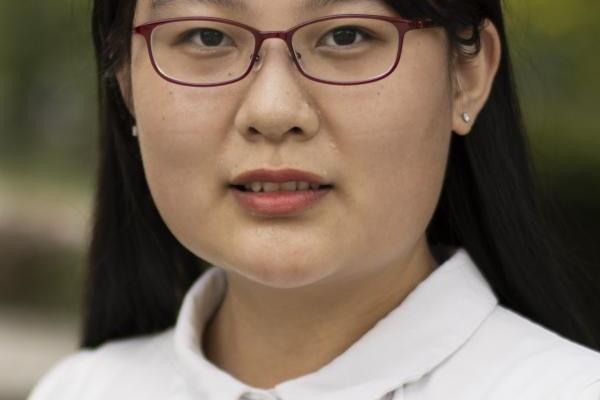
Dissertation Defense: Yuxuan Xin, Statistics PhD Candidate
Title: A Bayesian Semi-parametric Approach for Heterogeneous Causal Effect Estimation in Observational Studies And its Sensitivity Analysis
Abstract: Causal inference using observational data is important in fields where randomized experiments are infeasible. Traditional methods such as propensity score matching, weighting, and stratification rely on the assumption of ignorability and often struggle with nonlinear relationships and treatment effect heterogeneity. This dissertation addresses two core challenges of causal inference in observational studies: (1) flexibly estimating heterogeneous treatment effects in high-dimensional settings, and (2) assessing the sensitivity of causal conclusions to unmeasured confounding.
We propose a Bayesian semi-parametric framework called the Gaussian Process (GP) joint model that incorporates both propensity and prognostic scores as covariates and makes use of Gaussian Process (GP) priors. This approach enables flexible modeling of complex functions and estimation of both the conditional average treatment effect (CATE) and the population average treatment effect (PATE). To address unmeasured confounding, we extend this framework to develop the Gaussian Process Sensitivity Analysis (GPSA) model, which quantifies how causal estimates might vary under different assumptions about unmeasured confounding. We demonstrate the utility of the proposed models with data from the National Longitudinal Survey of Youth 1979 (NLSY79), estimating the causal effects of college attendance on outcomes, including income and fertility.
Zoom Link: https://osu.zoom.us/j/92439230386?pwd=IhRgMxcfF0dQfLAeJPdLz7hgJizePH.1