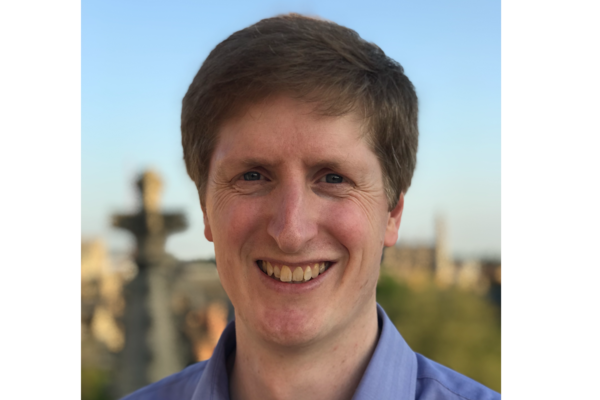
Title
USP: an independence test that improves on Pearson's chi-squared and the G-test
Speaker
Richard Samworth - University of Cambridge, Department of Pure Mathematics and Mathematical Statistics; Statistics Laboratory
Abstract
We introduce the U-Statistic Permutation (USP) test of independence in the context of discrete data displayed in a contingency table. Either Pearson's chi-squared test of independence, or the Generalised Likelihood Ratio test (G-test), are typically used for this task, but we argue that these tests have serious deficiencies, both in terms of their inability to control the size of the test, and their power properties. By contrast, the USP test is guaranteed to control the size of the test at the nominal level for all sample sizes, has no issues with small (or zero) cell counts, and is able to detect distributions that violate independence in only a minimal way. The test statistic is derived from a U-statistic estimator of a natural population measure of dependence, and we prove that this is the unique minimum variance unbiased estimator of this population quantity.
In the last one-third of the talk, I will show how this is a special case of a much more general methodology and theory for independence testing.