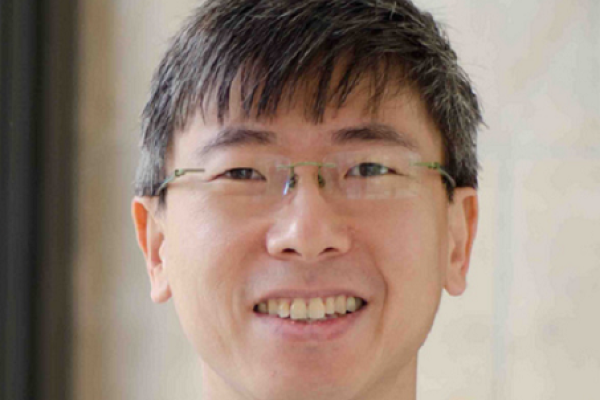
Speaker: Yee Whye Teh, Professor of the Department of Statistics, University of Oxford
Title: On recent advances in learning stochastic processes using neural networks
Abstract:
Bayesian nonparametrics is a popular area concerned with theory, methodology and practice of Bayesian inference and learning on large infinite-dimensional spaces of functions. Over the years, many classes of Bayesian nonparametric models have been studied, including Gaussian processes, Dirichlet processes and dependent Dirichlet processes. Much of the challenge of Bayesian nonparametrics comes down to how to properly define flexible and tractable distributions over functions (aka stochastic processes). Kolmogorov’s Consistency Theorem shows that these can be defined via consistent families of marginal distributions. Unfortunately, consistency is a very strong constraint and only particular families of stochastic processes can be constructed tractably in this way, limiting the flexibility and general applicability of Bayesian nonparametric models.
In recent years, there has been a number of interesting developments in the machine learning community, generally referred to as neural processes, which use neural networks to learn more flexible classes of stochastic processes by giving up on some of the limiting consistency properties. In this talk I will give an overview of these exciting developments, including some I have had the fortune to be involved in. I will relate these to popular ideas in machine learning, including meta-learning and deep generative models, and speculate on how we can recover the full range of consistency properties required to define proper stochastic processes.