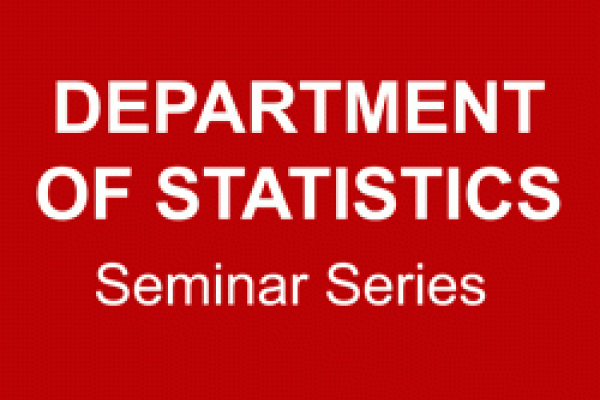
Title
Small-Variance Asymptotics for Large-Scale Learning
Speaker
Brian Kulis, The Ohio State University
Abstract
It is widely known that the Gaussian mixture model is related to k-means by “small-variance asymptotics”: as the covariances of the clusters shrink, the EM algorithm approaches the k-means algorithm and the negative log-likelihood approaches the k-means objective. Similar asymptotic connections exist for other machine learning models, including dimensionality reduction (probabilistic PCA becomes PCA), multiview learning (probabilistic CCA becomes CCA), and classification (a restricted Bayes optimal classifier becomes the SVM). The asymptotic non-probabilistic counterparts to the probabilistic models are almost always more scalable, and are typically easier to analyze, making them useful alternatives to the probabilistic models in many situations. I will explore how we can extend such asymptotics to a richer class of probabilistic models, with a focus on large-scale graphical models, Bayesian nonparametric models, and time-series data. I will develop the necessary mathematical tools needed for these extensions and will describe a framework for designing scalable optimization problems derived from the rich probabilistic models. Applications are diverse, and include topic modeling, network evolution and feature learning.