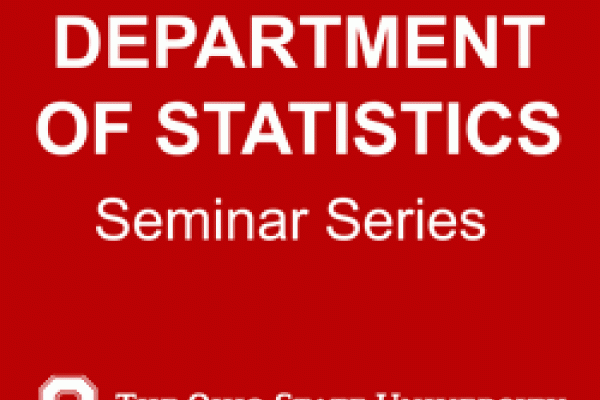
Title
Selecting an Estimator to Meet Multiple Goals in Hierarchical GLMs
Speaker
Dan Spitzner, Department of Statistics, University of North Carolina, Chapel Hill
Abstract
It is often the case that several evaluative criteria are available to assess the performance of a regression estimator, each tailored to a different purpose of the analysis. For instance, an epidemiological regression model for studying the health effects of airborne particulate matter will be presented, where the overall purpose of the analysis is to assist in regulatory decision-making. It is unclear, however, whether those decisions will be made based on knowledge of the parameters of the physical model, or its ability to make predictions. The answer is undoubtedly both; thus an analyst would want to select an estimator that performs well under at least two criteria. The problem is made precise in the context of decision theory where a set of loss functions, translated from the goals of the analysis, are the basis for measuring performance.
It is well known that in normal linear regression the ideas of ridge regression and shrinkage lead to improvements in squared-error loss over the maximum likelihood estimator. Hierarchical modeling, or empirical Bayes estimation, provides an automated mechanism for choosing a ridge parameter, and also a straightforward approach for extending ridge regression to generalized linear models (GLMs). Squared-error loss is replaced by entropy loss as an appropriate measure of performance. Several results will be presented that ultimately lead to a procedure for selecting an exponential family regression estimator to meet multiple goals: (i.) asymptotic expressions are derived for the difference in expected entropy loss between the maximum likelihood estimator and a Bayes rule; (ii.) a minimization argument is presented that suggests a general, very simple class of prior distributions from which candidate estimators are constructed; and, (iii.) risk diagnostics, based on the asymptotic expected loss calculations, are proposed as measures of improved performance. The procedure is demonstrated on the particulate matter data (using a Poisson regression model) to select an estimator that appears to outperform the maximum likelihood estimator under criteria for both parameter estimation and predictive ability.