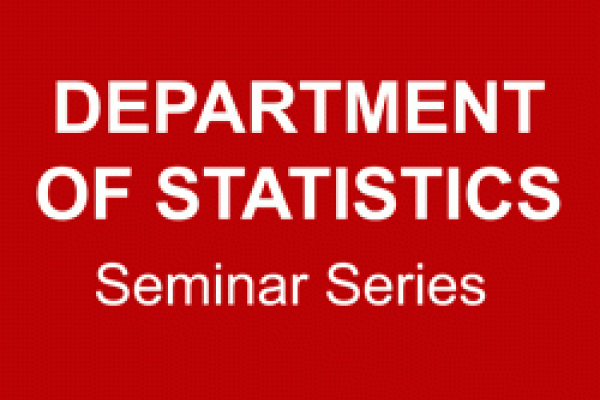
Title
Bayesian Compression in High-Dimensional Regression
Speaker
Rajarshi Guhaniyogi, Duke University
Abstract
As an alternative to variable selection or shrinkage in massive dimensional regression, we propose a novel idea to compress massive dimensional predictors to a lower dimension. Careful studies are carried out with several choices of compression matrices to understand their relative advantages and trade-offs. Compressing predictors with random compression dramatically reduces storage and computational bottlenecks, yielding accurate prediction when the predictors can be projected onto a low dimensional linear subspace with minimal loss of information about the response. As opposed to existing Bayesian dimensionality reduction approaches, the exact posterior distribution conditional on the compressed data is available analytically. This results in speeding up computation by many orders of magnitude while also bypassing robustness issues due to convergence and mixing problems with MCMC. Model averaging is used to reduce sensitivity to the random compression matrix, while accommodating uncertainty in the subspace dimension. Designed compression matrices additionally facilitate accurate lower dimensional subspace estimation along with good predictive inference. Novel modeling techniques are implemented to scale up these methods in presence of massive data or streaming data. Strong theoretical results are provided guaranteeing "optimal" convergence behavior for both these approaches. Practical performance relative to competitors is illustrated in extensive simulations and real data applications.