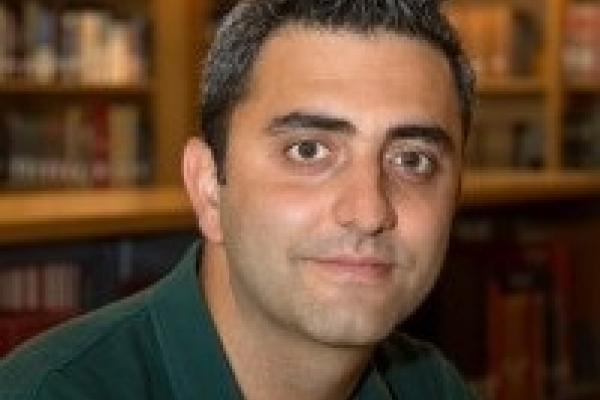
Title
A Convex Optimization Algorithm for Statistical Learning with Structured Sparsity
Speaker
Sam Davanloo Tajbakhsh, The Ohio State University, Department of Integrated Systems Engineering
Abstract
In some sparse statistical learning problems, the solution sparsity should follow some structures between the variables which are known a priori. Finding solutions that minimize loss functions of interest and also conform to such structures helps to obtain more interpretable statistical models. Exact solutions for such learning problems require Mixed Integer Programming (MIP) formulations which are generally, computationally, not easy to solve to optimality. Our study focuses on an approximate nonsmooth convex optimization formulation where the objective function is the sum of a loss function and a nonsmooth sparsity-inducing penalty. Since proximal methods plays a key role in solving nonsmooth optimization problems, we worked on a first-order optimization algorithm (i.e. uses up to the gradient information) with distributable subproblems to evaluate the proximal map of the sparsity-inducing penalty. In this talk, I will briefly discuss proximal methods for nonsmooth optimization, the nonsmooth sparsity-inducing penalty function, our proposed algorithm to find its proximal map, and the rate of convergence of the algorithm. I will finally show some numerical results supporting the proposed method.
Note: Seminars are free and open to the public. Reception to follow.