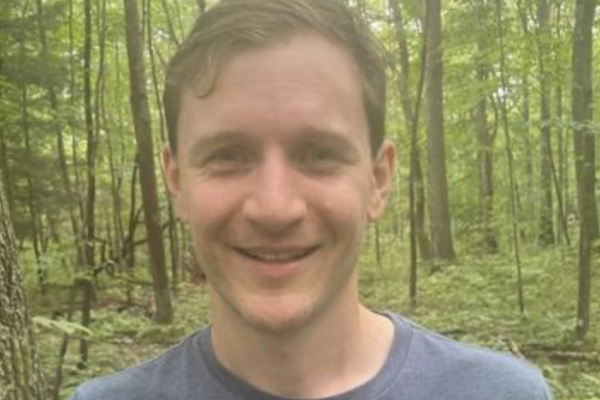
Speaker: Alden Green
Title: The High-Dimensional Asymptotics of Principal Components Regression
Abstract: We study principal components regression (PCR) in an asymptotic high-dimensional setting, where the number of data points is proportional to the dimension. We derive exact limiting formulas for estimation and prediction risk, which depend in a complicated manner on the eigenvalues of the population covariance, the alignment between the population PCs and the true signal, and the number of selected PCs. A key challenge in the high-dimensional setting stems from the fact that the sample covariance is an inconsistent estimate of its population counterpart, so that sample PCs may fail to fully capture potential latent low-dimensional structure in the data. We demonstrate this point through several case studies, including that of a spiked covariance model.