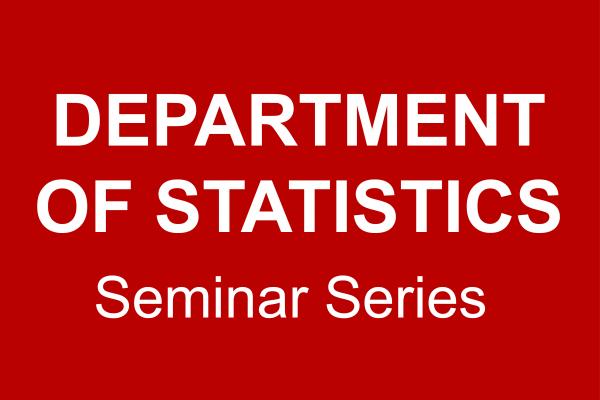
Title
Post-hoc Uncertainty Quantification for Remote Sensing Observing Systems
Speaker
Amy Braverman - Jet Propulsion Laboratory, NASA
Amy Braverman is Principal Statistician at the Jet Propulsion Laboratory, California Institute of Technology- a federally funded research and development center (FFRDC) operated by Caltech for NASA. She received her Ph.D. in Statistics from UCLA in 1999, and has been at JPL ever since. In the early part of her career she specialized in the analysis of massive remote sensing data sets for climate and Earth science, but over her 20 years at JPL she has developed methods and expertise in data fusion methods for heterogeneous remote sensing data sources, statistical methods for the evaluation of climate models, and most recently uncertainty quantification (UQ) especially remote sensing retrieval (inference) algorithms. In that area, she has led development of UQ methods and algorithms for a number of different missions including the Orbiting Carbon Observatory-2. Since 2013 Dr. Braverman has been a Technical Group Lead for Statistical Methods and Applications in the Instrument Software and Science Data Systems section of the Mission Systems and Operations Division. She is Fellow of the American Statistical Association, an Associate Editor for the journal Climatic Change and the SIAM/ASA Journal on Uncertainty Quantification.
Abstract
The ability of spaceborne remote sensing data to address important Earth and climate science problems rests crucially on how well the underlying geophysical quantities can be inferred from these observations. Remote sensing instruments measure parts of the electromagnetic spectrum and use computational algorithms to infer the unobserved true physical states. However, the accompanying uncertainties, if they are provided at all, are usually incomplete. There are many reasons why including but not limited to unknown physics, computational artifacts and compromises, unknown uncertainties in the inputs, and more.
In this talk I will describe a practical methodology for uncertainty quantification of physical state estimates derived from remote sensing observing systems. The method we propose combines Monte Carlo simulation experiments with statistical modeling to approximate conditional distributions of unknown true states given point estimates produced by imperfect operational algorithms. Our procedure is carried out post-hoc; that is, after the operational processing step because it is not feasible to redesign and rerun operational code. I demonstrate the procedure using four months of data from NASA's Orbiting Carbon Observatory-2 mission, and compare our results to those obtained by validation against data from the Total Carbon Column Observing Network where it exists.
Keywords: Uncertainty quantification, remote sensing, carbon cycle science.