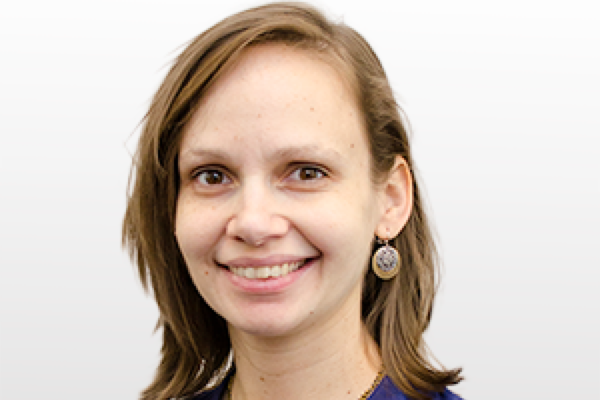
Speaker: Dena M. Asta, Department of Statistics, The Ohio State University
Title: Non-Parametric Manifold Learning
Abstract:
Graph Laplacians are certain matrices defined in terms of samples of random vectors drawn from a latent, unknown subspace of Euclidean space. The use of graph Laplacians to partially learn the geometry of a latent manifold is one of the dominant paradigms in machine learning. However, graph Laplacians as they are currently used can never completely recover the latent manifold in a non-parametric setting.
The goal of this talk is to show how graph Laplacians can actually be used to obtain a consistent estimator for intrinsic latent manifold distances between sample points, and in particular, a non-parametric but computable method of completely recovering the manifold. There are two main insights behind this method: 1) graph Laplacians can be regarded not just as linear operators but something we might call quadratic operators; and 2) a fundamental result from non-commutative geometry reformulates manifold distance purely in terms of such quadratic operators. This latter reformulation is a special case of the Kontorovich dual reformulation of Wasserstein distances known as Connes' Distance Formula.
This talk reviews existing work in manifold learning and does not assume any prior knowledge of manifolds, manifold distances, Connes' Distance Formula, or graph Laplacians.
Seminars are open to public.