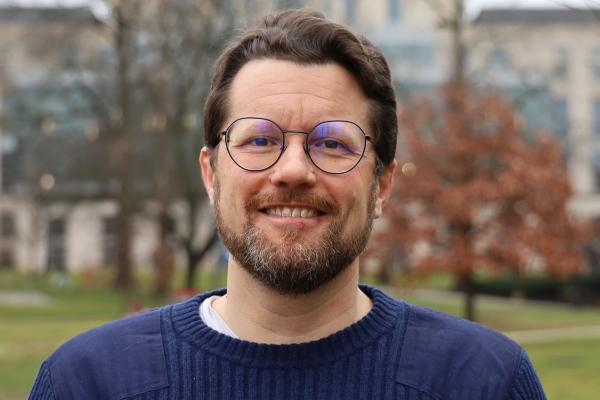
Speaker: Eben Kenah, Biostatistics, College of Public Health, OSU
Title: Potential Outcomes Approaches to Selection Bias
Abstract: Neither the structural approach to selection bias nor the traditional definition of selection bias can be stated explicitly in terms of the potential outcomes used to define causal effects. We propose a novel definition of selection bias in terms of potential outcomes that captures all selection bias under the structural approach and under the traditional definition of selection bias. It is nonparametric, and it can be analyzed using single-world intervention graphs (SWIGs) both under and away from the null hypothesis. It allows the simultaneous analysis of confounding and selection bias, explicitly links the selection of study participants to the estimation of causal effects, and can be adapted to handle selection bias in descriptive epidemiology. It provides a novel perspective on the mechanisms that can generate selection bias, and it simplifies the analysis of matched studies and case-cohort studies. Finally, I discuss a simplification and extension of this approach being developed by Patrick Schnell that uses a type of causal graph that we call partial SWIGs.