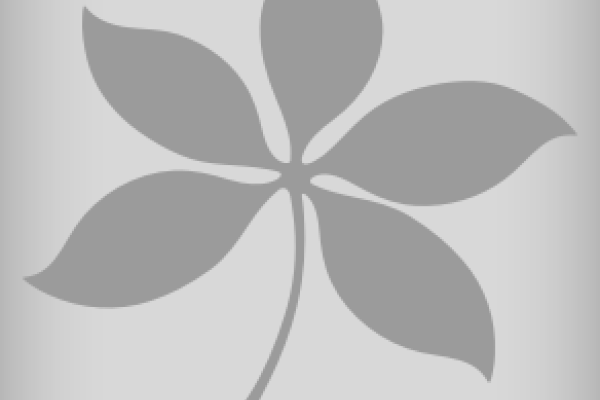
Speaker: Evan Rosenman, Postdoctoral Scholar, Harvard Data Science Initiative
Title: Shrinkage Estimation for Causal Inference and Experimental Design
Abstract: How can observational data be used to improve the design and analysis of randomized controlled trials (RCTs)? We first consider how to develop estimators to merge causal effect estimates obtained from observational and experimental datasets, when the two data sources measure the same treatment. To do so, we extend results from the Stein shrinkage literature. We propose a generic "recipe" for deriving shrinkage estimators, making use of a generalized unbiased risk estimate. Using this procedure, we develop two new estimators and prove finite sample conditions under which they have lower risk than an estimator using only experimental data. Next, we consider how these estimators might contribute to more efficient designs for prospective randomized trials. We show that the risk of a shrinkage estimator can be computed efficiently via numerical integration. We then propose algorithms for determining the experimental design -- that is, the best allocation of units to strata -- by optimizing over this computable shrinker risk.
Note: Seminars are free and open to the public. Reception to follow.