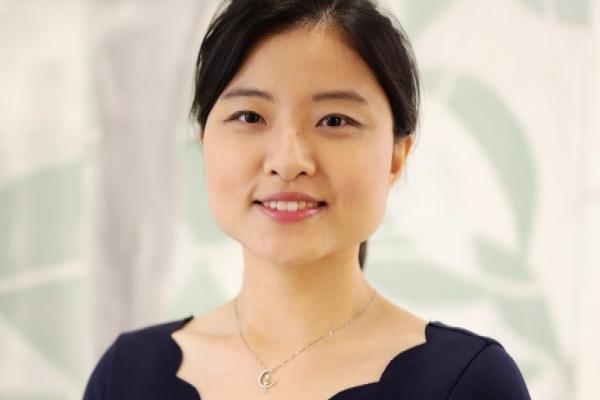
Speaker: Fan Bu, Postdoctoral Scholar in Department of Biostatistics, University of California – Los Angeles
Title: Statistical Methods for Observational Data on Infectious Diseases
Abstract: Emerging modern datasets in public health call for development of innovative statistical methods that can leverage complex real-world data settings. We first discuss a stochastic epidemic model that incorporates contact tracing data to make inference about transmission dynamics on an adaptive contact network. An efficient data-augmented inference scheme is designed to accommodate partially epidemic observations. This networked epidemic model allows flexible extensions to account for individual heterogeneity, disease latency and social interventions to help bring new epidemiological insights. We then discuss a collaborative work with the US FDA to improve post-market vaccine safety surveillance procedures. We propose a Bayesian statistical framework to tackle the challenge of sequentially analyzing observational healthcare data to detect vaccine adverse events. This new framework is substantially more flexible as it does not require a pre-specified analysis schedule in the standard procedure. It also adaptively corrects for bias in observational healthcare data to improve the quality of data-driven decision-making by reducing decision error.
Note: Seminars are free and open to the public. Reception to follow.