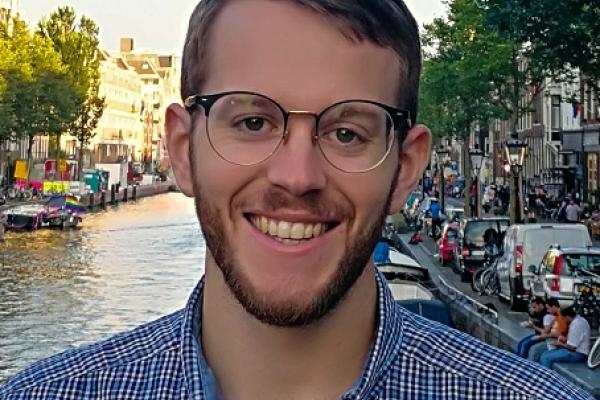
Speaker: Jared Huling, Assistant Professor, Biostatistics, University of Minnesota
Title: Transportability of Principal Causal Effects
Abstract: Recent research in causal inference has made important progress in addressing challenges to the external validity of trial findings. Such methods weight trial participant data to more closely resemble the distribution of effect-modifying covariates in a well-defined target population. In the presence of participant non-adherence to study medication, these methods effectively transport an intention-to-treat effect that averages over heterogeneous compliance behaviors. In this paper, we develop a principal stratification framework to identify causal effects conditioning on both compliance behavior and membership in the target population. We also develop non-parametric efficiency theory for and construct efficient estimators of such "transported" principal causal effects and characterize their finite-sample performance in simulation experiments. We illustrate our methods with a study of the effect of a health system intervention studied in a randomized trial in Camden, New Jersey with the aim of determining whether the intervention shows promise among high engagers with the intervention in a Midwestern health system. While this work focuses on treatment non-adherence, our framework is applicable to a broad class of estimands that target effects in clinically-relevant, possibly latent subsets of a target population defined by post-randomization events, such as effects among survivors.
Short Bio: Jared Huling is an Assistant Professor of Biostatistics at the University of Minnesota. His research interests focus on the development of causal inference, precision medicine, and statistical/machine learning methodology for the analysis of complex observational studies. He is particularly interested in methods for generalizability, combining different data sources, and addressing various forms of population and effect heterogeneity with the aim of improving patient health outcomes. His work in this area has involved applications in health system risk modeling, in personalizing health system intervention enrollment decisions, and comparative effectiveness using Electronic Medical Records.