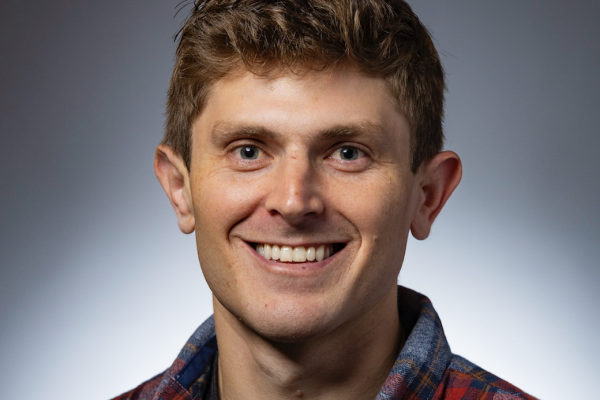
Speaker: Jordan Bryan
Title: Precise feasible linear least squares estimates for non-isotropic error
Abstract: Linear least squares theory is old, yet it is stubbornly relevant to many applications in modern natural science. Using this theory, I both motivate the design of and describe the properties of certain regression estimates that are simple to compute and are free of tuning parameters. In the setting of a linear mixing model for fluorescence spectra, I show that one such estimate, which is computable using the tools of ordinary least squares (OLS), is a close approximation to the best linear unbiased estimate (BLUE) of the mixing coefficients. In the context of the heteroscedastic linear model, I propose an M-estimate based on the t-distribution and demonstrate that it satisfies a favorable oracle property with respect to the BLUE and OLS estimates. I then discuss extensions to these investigations in relation to my research priorities for the near future.