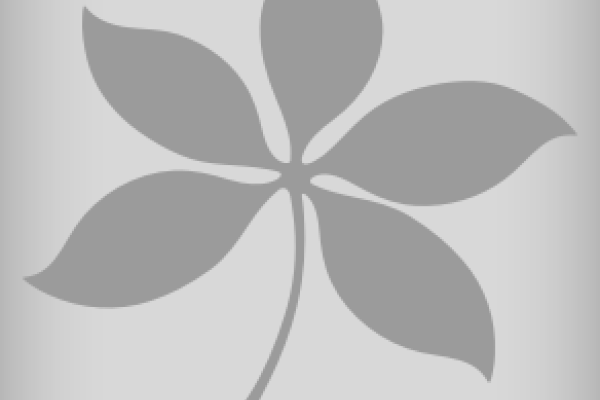
Speaker: Jose Angel Sanchez Gomez, PhD candidate in Statistics, University of North Carolina, Chapel Hill
Title: Detecting hub variables in large Gaussian graphical models
Abstract:
In modern scientific applications, identifying small sets of variables in a dataset with a strong influence over the rest is often vital. For example, when studying the gene-expression levels of cancer patients, estimating the most influential genes can be a first step towards understanding underlying gene dynamics and proposing new treatments. A popular approach to representing variable influence is through a Gaussian graphical model (GGM), where each variable corresponds to a node, and a link between two nodes represents relationships among pairs of variables. In a GGM, influential variables correspond to nodes with a high degree of connectivity, also known as hub variables.
In this talk, I share new methods for estimating hub variables in GGMs. To this end, we establish a connection between the presence of hubs in a GGM and the concentration of principal component vectors on the hub variables. We provide probabilistic guarantees of convergence for our method, even in high-dimensional data where the number of variables can be arbitrarily large. I will also discuss an applications of this new method to a prostate cancer gene-expression dataset, through which we detect several hub genes with close connections to tumor development.
Note: Seminars are free and open to the public. Reception to follow.