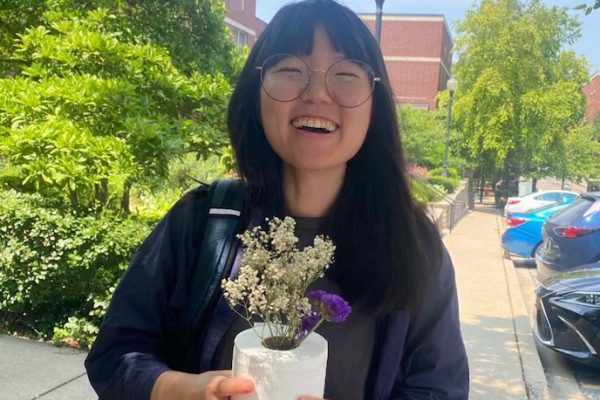
Speaker: Dr. Jungeum Kim
Title: Conformal Tree
Abstract: This talk is about uncertainty Quantification for generative AI. It will focus on conformal prediction in contemporary applications, where a black-box model has been trained on data that are not accessible to the user. Mirroring split-conformal inference, we develop a wrapper around black-box algorithms to calibrate conformity scores. This calibration is local and proceeds in two stages: first, adaptively partitioning the predictor space into groups and then calibrating sectionally group by group. Adaptive partitioning (self-grouping) is achieved by fitting a robust regression tree to the conformity scores on the calibration set. Applications include uncertainty quantification for GPT-4 predictions, such as conformalizing skin disease diagnoses based on self-reported symptoms.