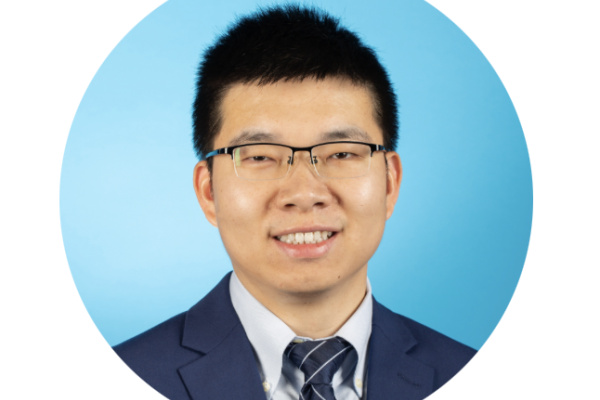
February 11, 2025
3:00PM
-
4:00PM
EA 170
Add to Calendar
2025-02-11 16:00:00
2025-02-11 17:00:00
Seminar Series: Kai Tan
Speaker: Kai TanTitle: Estimating Generalization Error for Iterative Algorithms in High-Dimensional Regression Abstract: In the first part of the talk, I will investigate the generalization error of iterates from iterative algorithms in high-dimensional linear regression. The proposed estimators apply to Gradient Descent, Proximal Gradient Descent, and accelerated methods like FISTA. These estimators are consistent under Gaussian designs and enable the selection of the optimal iteration when the generalization error follows a U-shaped pattern. Simulations on synthetic data demonstrate the practical utility of these methods. In the second part of the talk, I will focus on the generalization performance of iterates obtained by Stochastic Gradient Descent (SGD), and their proximal variants in high-dimensional robust regression problems. I will introduce estimators that can precisely track the generalization error of the iterates along the trajectory of the iterative algorithm. These estimators are shown to be consistent under mild conditions that allow the noise to have infinite variance. Extensive simulations confirm the effectiveness of the proposed generalization error estimators.
EA 170
OSU ASC Drupal 8
ascwebservices@osu.edu
America/New_York
public
Date Range
2025-02-11 15:00:00
2025-02-11 16:00:00
Seminar Series: Kai Tan
Speaker: Kai TanTitle: Estimating Generalization Error for Iterative Algorithms in High-Dimensional Regression Abstract: In the first part of the talk, I will investigate the generalization error of iterates from iterative algorithms in high-dimensional linear regression. The proposed estimators apply to Gradient Descent, Proximal Gradient Descent, and accelerated methods like FISTA. These estimators are consistent under Gaussian designs and enable the selection of the optimal iteration when the generalization error follows a U-shaped pattern. Simulations on synthetic data demonstrate the practical utility of these methods. In the second part of the talk, I will focus on the generalization performance of iterates obtained by Stochastic Gradient Descent (SGD), and their proximal variants in high-dimensional robust regression problems. I will introduce estimators that can precisely track the generalization error of the iterates along the trajectory of the iterative algorithm. These estimators are shown to be consistent under mild conditions that allow the noise to have infinite variance. Extensive simulations confirm the effectiveness of the proposed generalization error estimators.
EA 170
America/New_York
public
Speaker: Kai Tan
Title: Estimating Generalization Error for Iterative Algorithms in High-Dimensional Regression
Abstract: In the first part of the talk, I will investigate the generalization error of iterates from iterative algorithms in high-dimensional linear regression. The proposed estimators apply to Gradient Descent, Proximal Gradient Descent, and accelerated methods like FISTA. These estimators are consistent under Gaussian designs and enable the selection of the optimal iteration when the generalization error follows a U-shaped pattern. Simulations on synthetic data demonstrate the practical utility of these methods.
In the second part of the talk, I will focus on the generalization performance of iterates obtained by Stochastic Gradient Descent (SGD), and their proximal variants in high-dimensional robust regression problems. I will introduce estimators that can precisely track the generalization error of the iterates along the trajectory of the iterative algorithm. These estimators are shown to be consistent under mild conditions that allow the noise to have infinite variance. Extensive simulations confirm the effectiveness of the proposed generalization error estimators.