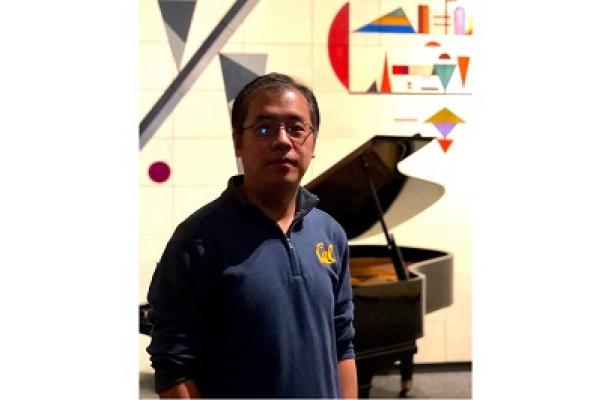
Title
Multimodal Neuroimaging Analysis
Speaker
Lexin Li - University of California at Berkeley, Department of Biostatistics and Epidemiology & Helen Wills Neuroscience Institute
Abstract
Multimodal neuroimaging is now becoming a norm in neuroscience research. It utilizes different physical and physiological sensitivities of imaging scanners and technologies, and acquires different types of brain images for a common set of experimental subjects. It measures distinct brain characteristics, ranging from brain structure and function to numerous chemical constituents, and produces a variety of imaging modalities, including anatomical magnetic resonance imaging (MRI), functional magnetic resonance imaging (fMRI), positron emission tomography (PET), among many others. Multimodal neuroimaging analysis aggregates such diverse but often complementary information, and borrows strength across different modalities to render an integrated data resolution that would otherwise not be available with any single data type. In this talk, we examine a number of statistical problems and present two case studies in multimodal neuroimaging analysis. One is generalized liquid association analysis, and the other is integrative factor regression inference.