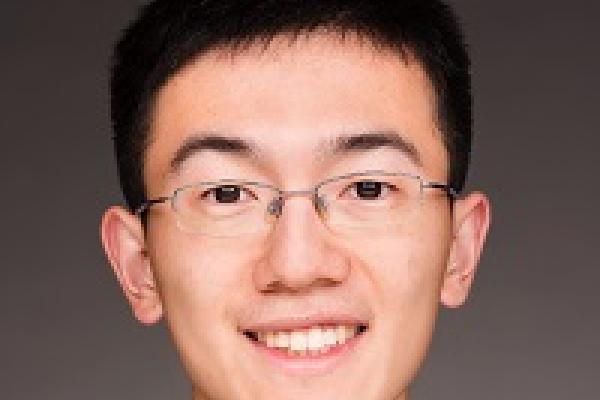
Title
The cost of privacy: optimal rates of convergence for parameter estimation with differential privacy
*Please mute upon entry
Speaker
Linjun Zhang, Rutgers University, Department of Statistics
Abstract
Privacy-preserving data analysis is a rising challenge in contemporary statistics, as the privacy guarantees of statistical methods are often achieved at the expense of accuracy. In this paper, we investigate the tradeoff between statistical accuracy and privacy in generalized linear models, under both the classical low- dimensional and modern high-dimensional settings. A primary focus is to establish minimax optimality for statistical estimation with the (\epsilon, \delta)-differential privacy constraint. To this end, we find that classical lower bound arguments fail to yield sharp results, and new technical tools are called for.
Inspired by the theoretical computer science literature on “tracing adversaries”, we formulate a general lower bound argument for minimax risks with differential privacy constraints, and apply this argument to high-dimensional generalized linear models. We also design computationally efficient algorithms that attain the minimax lower bounds up to logarithmic factors. In particular, a novel private iterative hard thresholding pursuit algorithm is proposed, based on a privately truncated version of stochastic gradient descent. The numerical performance of these algorithms is demonstrated by simulation studies and applications to real data containing sensitive information, for which privacy-preserving statistical methods are necessary.