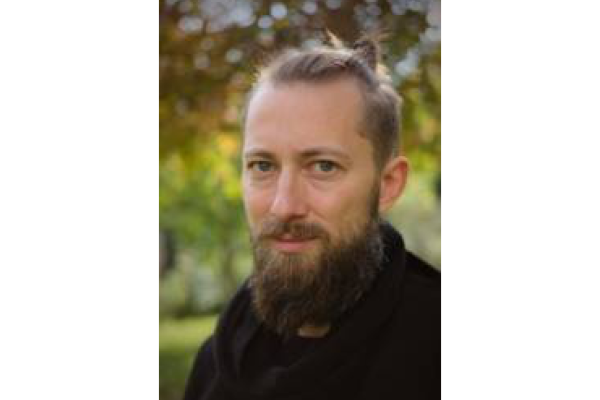
Speaker: Paul Wiemann
Title: Efficient Gaussian Process Inference with Vecchia-type Approximations for Non-Gaussian Data
Abstract: Gaussian Processes (GPs) are probabilistic models for functions commonly used in statistics and machine learning. Despite their popularity in applications like spatial data analysis, GPs are computationally expensive as they rely on dense covariate matrices. This makes direct applications of GPs infeasible for large datasets. The Vecchia approximation offers a solution by approximating the joint density with a product of conditional distribution with reduced conditioning sets. This approximation implies a sparse Cholesky factor of the precision matrix, allowing for fast and scalable GP inference. We discuss the applications of Vecchia-type approximations in environmental statistics. Specifically, we cast the problem of learning the distribution of a multivariate spatial field from a small number of replicates into a series of independent GP regression tasks with Gaussian errors. Due to the potential nonlinearity in the conditional means, the joint distribution modeled can be non-Gaussian, and the methodology is well-suited to be applied to the output of climate models. Additionally, leveraging ideas from machine learning, we demonstrate how Vecchia-type approximations facilitate the use of scalable and highly adaptable variational inference targeting the non-Gaussian posterior of a latent continuous function or field.
Note: Seminars are free and open to the public. Reception to follow.