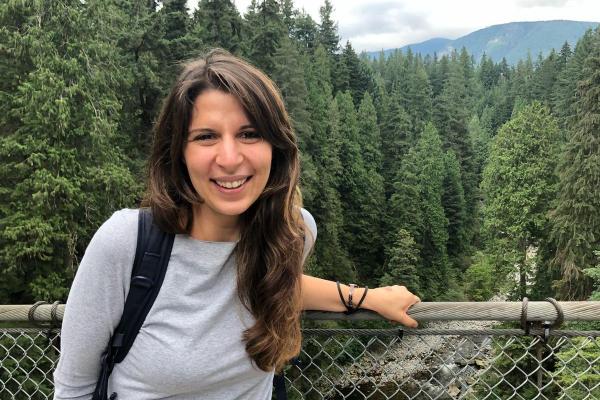
(Meeting link announced in email to OSU statistics seminar mail list recipients.)
Title
Handling model uncertainties via informative Goodness-of-Fit
Speaker
Sara Algeri, University of Minnesota, School of Statistics
Abstract
When searching for signals of new astrophysical phenomena, astrophysicists have to account for several sources of non-random uncertainties which can dramatically compromise the sensitivity of the experiment under study. Among these, model uncertainty arising from background mismodeling is particularly dangerous and can easily lead to highly misleading results. Specifically, overestimating the background distribution in the signal region increases the chances of falsely rejecting the hypothesis that the new source is present. Conversely, underestimating the background outside the signal region leads to an artificially enhanced sensitivity and a higher likelihood of claiming a false discovery. The aim of this work is to provide a self-contained framework to perform modeling, estimation, and inference under background mismodeling. The method proposed allows incorporating the (partial) scientific knowledge available on the background distribution, and provides a data-updated version of it in a purely nonparametric fashion, and thus, without requiring the specification of prior distributions. If a calibration (or control regions) is available, the solution discussed does not require the specification of a model for the signal, however when available, it allows to further improve the accuracy of the analysis and to detect additional and unexpected signal sources.