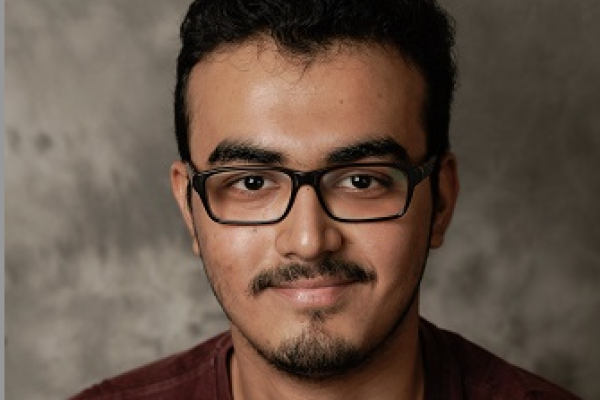
Speaker: Shounak Chattopadyay
Title: Leveraging the blessing of dimensions for scalable Bayesian inference on covariance matrices
Abstract: Bayesian factor analysis provides an elegant approach for probabilistic inference on high-dimensional covariance matrices, decomposing the covariance as a sum of a low-rank and diagonal matrix. Posterior computation in such models typically involves Markov chain Monte Carlo (MCMC) algorithms such as Gibbs sampling steps that alternately update the latent factors, factor loadings, and residual variances. In this talk, we exploit a remarkable blessing of dimensionality phenomenon to develop a provably accurate approximate-posterior for the covariance matrix, completely bypassing the need for MCMC via an embarrassingly parallel computational framework.
The proposed Factor Analysis with BLEssing of dimensionality (FABLE) approach relies on a first-stage singular value decomposition to estimate the latent factors, then defines a jointly conjugate prior for the loadings and residual variances. The accuracy of the resulting approximate-posterior for the covariance improves with increasing dimensionality. FABLE has excellent performance in high-dimensional covariance matrix estimation, including producing well-calibrated credible intervals, both theoretically and through simulation experiments. We also demonstrate the strength of our approach in terms of accurate inference and computational efficiency by applying it to a gene expression data set.
This talk will also briefly provide other examples of novel computational and probabilistic modeling frameworks to analyze complex data, with interesting future directions.