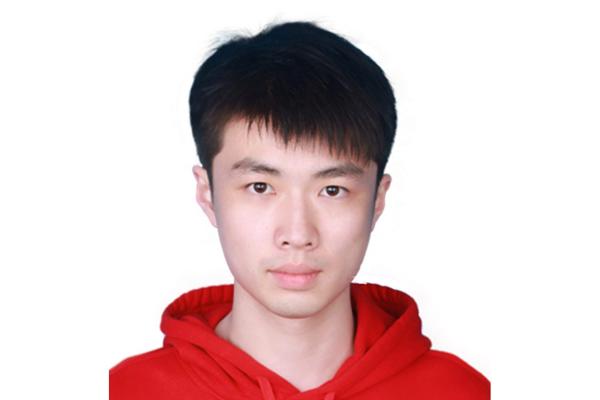
Speaker: Siyu Heng, PhD Candidate, University of Pennsylvania
Title: Removing Strong Data Assumptions in Causal Inference via Large-Scale Optimization
Abstract:
Causal inference seeks to study the causal effects of various human interventions and has deeply influenced decision making in public health research and biomedical research. However, many traditional and newly developed causal inference methods rely on strong assumptions about the data distribution and/or data collection procedure, and if those assumptions do not hold, they may either be inapplicable or suffer from low statistical power.
In this talk, we use two examples to illustrate how large-scale optimization can aid in conducting valid and powerful causal inference without imposing strong data assumptions, and how these methodological advances provide benefits for studying health issues for communities, especially low-income communities.
If time permits, we also discuss some ongoing projects and future directions in optimization-based methods for causal inference.