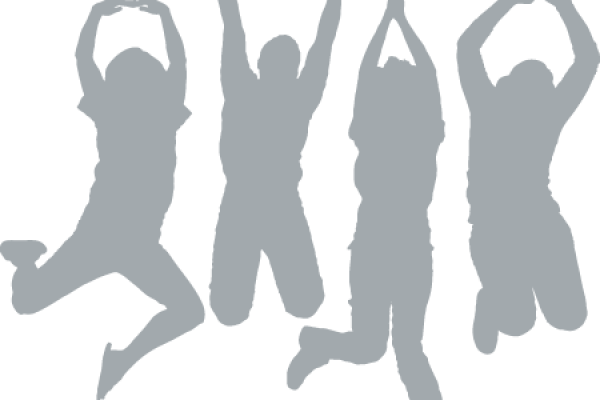
Speaker: Hyoin An
Title: Bayesian Quantile Regression via Dependent Quantile Pyramids
Abstract: The simultaneous estimation of multiple quantile regression (QR) curves has gained increasing attention as an alternative to mean regression, yet it remains challenging. We develop a new class of stochastic processes, a process of dependent quantile pyramids (DQPs). This class is applied to build a flexible simultaneous QR model that falls within the Bayesian nonparametric framework. The process of DQPs generalizes the quantile pyramid, a model for a single set of quantiles without a covariate. The generalization replaces each scalar variate in the quantile pyramid with a stochastic process whose index set is a covariate space. The resulting model is a distribution-valued stochastic process which provides a nonparametric distribution at each value of the covariate. We rigorously establish the existence of the model. Simulation studies document the performance of our approach. An application to cyclone intensity data is presented.
Speaker: Meijia Shao
Title: Network two-sample test
Abstract: : Two-sample hypothesis testing for network comparison presents many significant challenges, including: leveraging repeated network observations and known node registration, but without requiring them to operate; relaxing strong structural assumptions; achieving finite-sample higher-order accuracy; handling different network sizes and sparsity levels; fast computation and memory parsimony; controlling false discovery rate (FDR) in multiple testing; and theoretical understandings, particularly regarding finite-sample accuracy and minimax optimality. In this paper, we develop a comprehensive toolbox, featuring a novel main method and its variants, all accompanied by strong theoretical guarantees, to address these challenges. Our method outperforms existing tools in speed and accuracy, and it is proved power-optimal. Our algorithms are user-friendly and versatile in handling various data structures (single or repeated network observations; known or unknown node registration). We also develop an innovative framework for offline hashing and fast querying as a very useful tool for large network databases. We showcase the effectiveness of our method through comprehensive simulations and applications to two real-world datasets, which revealed intriguing new structures.
Note: Seminars are free and open to the public. Reception to follow.