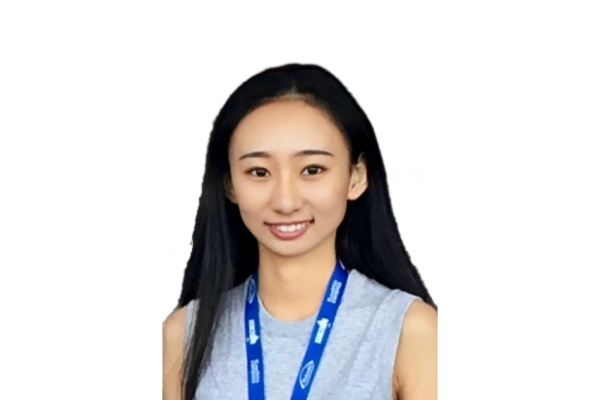
Speaker: Xiaoxuan Cai, Postdoc, Columbia University
Title: Causal Inference under interference: new methods and their application to infectious disease and mobile health.
Abstract:
Causal identification under interference when outcomes are not revealed independently has been a challenging subject to study in epidemiology, public health, political science, and medical research. The causal evaluation of infectious disease interventions (e.g., vaccination) in an interactive population suffers from “interference” across subjects as the outcome of interest – infection – is transmissible between individuals. In my talk, I will present my recent work on a general stochastic model of infectious disease transmission in continuous time, and natural definitions of causal estimands for individual-level direct and indirect vaccine effects, which involve multiple time scales and permit regression adjustment for variables associated with susceptibility to disease, and infectiousness once infected. Mobile health is another area suffering from “interference” across time points, as the collected time series data of outcomes highly depend on previous outcomes and exposures. Motivated by a smartphone observational study – the Bipolar Longitudinal Study, we propose a novel dynamic imputation method for N-of-1 studies that accommodates the non-stationarity of time series when treatment effect may change over. We also propose a set of novel causal estimands for (potentially non-stationary) multivariate time series in N-of-1 studies to systematically summarize how time-varying exposures affect outcomes in the short and long term and derive their identification via the g-formula in the presence of exposure- and outcome-covariate feedbacks.