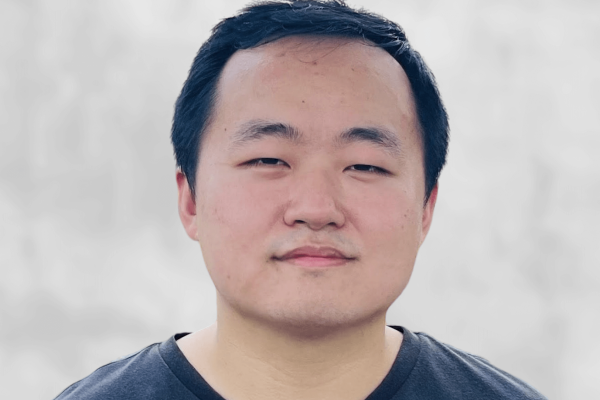
Speaker: Dr. Yao Zhang
Title: Posterior Conformal Prediction
Abstract: Conformal prediction is a popular technique for constructing prediction intervals with distribution-free coverage guarantees. The coverage is marginal, holding on average over the entire population but not necessarily for any specific subgroup. In this talk, I will introduce a new method, posterior conformal prediction (PCP), which generates prediction intervals with both marginal and approximate conditional coverage for clusters (or subgroups) naturally discovered in the data. PCP achieves these guarantees by modelling the conditional conformity score distribution as a mixture of cluster distributions. Compared to other methods with approximate conditional coverage, this approach produces tighter intervals, particularly when the test data is drawn from clusters that are well represented in the validation data. PCP can also be applied to guarantee conditional coverage on user-specified subgroups, in which case it achieves robust coverage on smaller subgroups within the specified subgroups. In classification, the theory underlying PCP allows for adjusting the coverage level based on the classifier’s confidence, achieving significantly smaller sets than standard conformal prediction sets. Experiments demonstrate the performance of PCP on diverse datasets from socio-economic, scientific and healthcare applications.