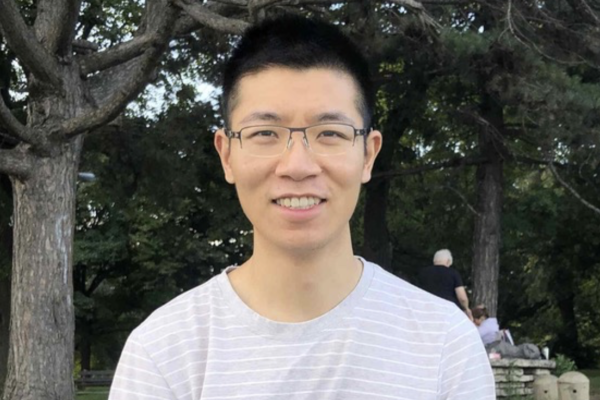
Speaker: Yuetian Luo
Title: Challenges and Opportunities in Assumption-free and Robust Inference
Abstract: With the growing application of data science to complex high-stakes tasks, ensuring the reliability of statistical inference methods has become increasingly critical. This talk considers two key challenges to achieving this goal: model misspecification and data corruption, highlighting their associated difficulties and potential solutions. In the first part, we investigate the problem of distribution-free algorithm risk evaluation, uncovering fundamental limitations for answering these questions with limited amounts of data. To navigate the challenge, we will also discuss how incorporating an assumption about algorithmic stability might help. The second part focuses on constructing robust confidence intervals in the presence of arbitrary data contamination. We show that when the proportion of contamination is unknown, uncertainty quantification incurs a substantial cost, resulting in optimal robust confidence intervals that must be significantly wider.