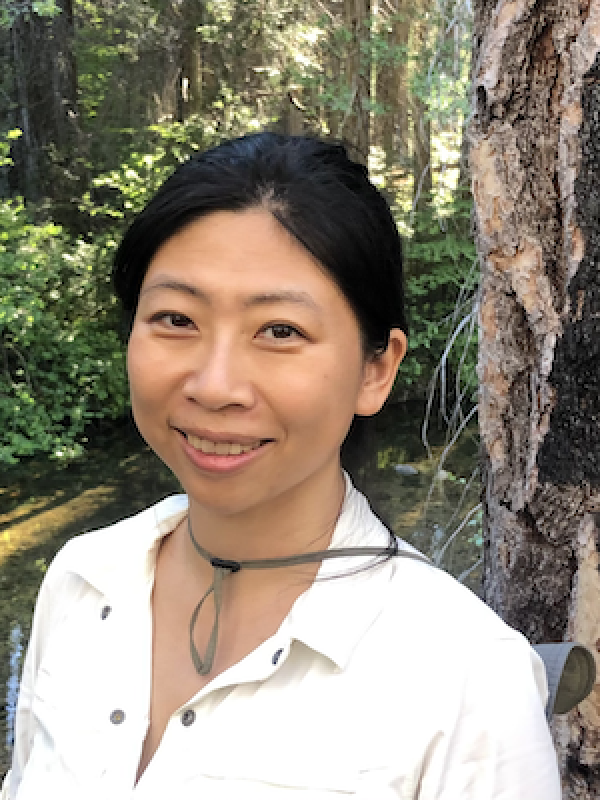
Areas of Expertise
- Use of auxiliary Information
- Cost-effective study design
- Z-estimation
- Proximal causal inference
- Casual inference with environmental data
Education
- PhD: Department of Biostatistics, University of Washington, Seattle, 2014
- BS: Department of Biochemistry, University of Hong Kong, 2006
Kate Hu joined the Department of Statistics in 2024, following her roles as Head of Data Science at Aclima, Inc. and Senior Data Scientist at Climate LLC, both climate-tech companies based in San Francisco. She earned her PhD from the University of Washington, Seattle, where her research focused on semiparametric inference, empirical processes, two-phase sampling, and the use of auxiliary information. During her postdoctoral research at Harvard University, she explored proximal causal inference with environmental data.
Her current statistical research interests include
- causal inference with environmental data
- proximal causal inference
- use auxiliary information to improve statistical inference
- study design
- automating asymptotic analysis
Her current applied statistical research interests include
- precision agriculture
- epidemiology
- climate hazards
- design environmental monitoring network
For students who are looking for reading courses, RA positions, dissertation topics, and research projects, you are welcome to contact me if you are interested in
- causal inference with time series data
- causal inference with spatial data
- causal inference with continuous treatment
- selection bias/confounding bias
- use proxies to adjust for bias (proximal causal inference)
- smart use of auxiliary information
- automating asymptotic analysis
- any applied statistical research topics listed above
For master or undergrad students, an additional topic is
- statistics and decision-making
My research style is problem-drive, which means we will focus on together formulating and solving a meaningful problem. The choice of approaches and tools—whether Bayesian, frequentist, machine learning, or semiparametric models—depends on the specific problem at hand.